The Rich Language of Probability: A Conversation with Luciano Pomatto
What is the chance that it will rain tomorrow? Weather reports, like many other predictions, give us probabilities of different events—a 50 percent chance of rain or a 90 percent chance of sunshine. Probabilistic statements allow scientists to quantify the degree of uncertainty about events. However, according to Assistant Professor of Economics Luciano Pomatto, probabilistic statements also allow people to hide ignorance and feign knowledge. We sat down with Pomatto, who recently came to Caltech from a postdoctoral fellowship at Yale, to discuss the limits of probability and prediction.
How is it possible to "hide" behind probabilistic language?
I think this is best described with an example. Let's say we want to know the probability that in the next five years the sea level in Florida will rise more than one foot. If I tell you there is a 99.99 percent chance that this will happen, it would be very easy to see, in five years, if I was correct or not. If I tell you the chance is very slim, you are also able to easily find out if I'm correct or not in five years.
Now, let's say that I tell you that I did all the research, and I have determined that the probability is 50 percent. Even though 50 percent is more imprecise than 99.99 percent, if correct, it's still a valuable piece of knowledge—it would help you compute the correct expected return of an investment in the Miami area, for example.
The issue, however, is that it is impossible to test after the fact whether or not a statement such as "the sea rise will happen with 50 percent probability" is factually correct. There is, according to the forecast, an equal probability that such an event will or will not happen—so the prediction cannot be discredited by any single observation. This is a simple but fundamental difficulty. We only get to see if the event occurred or not, not whether it was likely or unlikely.
Thus, one can hide behind this language and pretend to be knowledgeable about the topic.
How does this relate to your current research?
Basic statistical intuition tells us that inference requires repeated observations. If a forecaster can be evaluated on the basis of many consecutive predictions, then it is natural to expect we should be able to tell whether or not such a forecaster is competent. For example, a weatherman who every morning announces a 50 percent probability of rain will soon be discredited unless it actually rains, on average, every other day.
Recently, economists have begun to study this problem more systematically. The main conclusion is that it is surprisingly hard to construct statistical tests that can distinguish between a true expert who knows the actual odds governing the problem from someone who is simply pretending to be knowledgeable. This is true regardless of the number of predictions on which forecasters are tested.
This strand of research started by examining some of the tests that are actually used in practice to evaluate forecasts. What has been shown is that in order to pass some of these tests, you don't really need to know anything about the phenomenon you are asked to predict. What you need to know is the exact test by which you are going to be evaluated, which has been a surprising finding.
In my own research, I develop a statistical test that can, under some assumptions, distinguish between informed and uninformed forecasters. The test is based on a simple intuition: it compares the predictions of the forecaster to the predictions of a fictitious automated forecaster created by the test. This fictitious forecaster represents a benchmark that a predictor must beat in order to qualify as knowledgeable. While the test is relatively straightforward to implement, the difficulty is in constructing the "right" fictitious forecaster. A benchmark that is too strict may discourage even honest forecasters from speaking their mind. A benchmark that is too loose would allow someone who is strategic but uninformed to pass the test.
What got you interested in this particular research?
I am very interested in how people think in situations where there is uncertainty. This particular line of research tackles a basic problem: What are the consequences of accepting probability as a language for making predictions about the future? Can any statement made in probabilistic terms be tested empirically in the same way the laws, say, of classical mechanics can be tested empirically? I find it a fascinating question.
What led you to becoming an economist?
I grew up in Northern Italy, and for most of my teenage years I was mainly interested in the humanities—until I attended a course in microeconomics, a branch of economics studying the behavior of individuals. Economics studies problems that are rooted in everyday reality: How are prices formed? How do people trade? How should we tax income? What fascinated me at the time is how economists strive to identify, starting from these specific questions, a small number of universal principles.
After my bachelor's degree in Italy, I got my PhD from Northwestern University in economics, where I worked on the problem of testing probabilistic predictions as well as other problems related to risk and uncertainty.
What do you like to do in your free time?
I like to watch movies, read, hike, and spend time in museums. I like to do things that give me some time to think.
For example, I recently went to the Getty Museum, and it was a very interesting experience. I'm really interested in understanding how creative people—like designers, architects, or writers—think about their work, what motivates them. I find that creative people are not so different from academics.
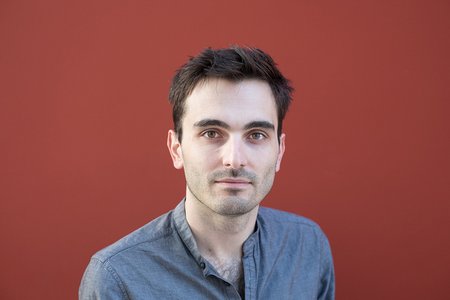
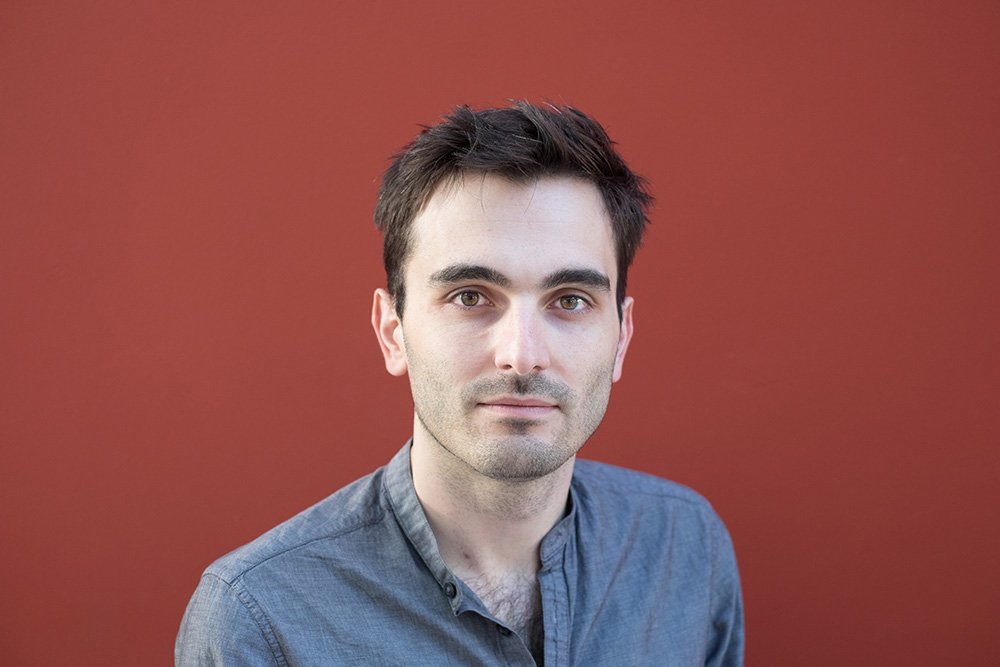